[Summary: a critical look at the UK’s Algorithmic Transparency Standard]
I was interested to see announcements today that the UK has released an ‘Algorithmic Transparency Standard’ in response to calls recommendations from the Centre for Data Ethics and Innovation (CDEI) “that the UK government should place a mandatory transparency obligation on public sector organisations using algorithms to support significant decisions affecting individuals”, and commitments in the National Data Strategy to “explore appropriate and effective mechanisms to deliver more transparency on the use of algorithmic assisted decision making within the public sector”and National AI Strategy to “Develop a cross-government standard for algorithmic transparency.”. The announcement is framed as “strengthening the UK’s position as a world leader in AI governance”, yet, at a closer look, there’s good reason to hold out judgement on whether it can deliver this until we see what implementation looks like.
Here’s a rapid critique based purely on reading the online documentation I could find. (And, as with most that I write, this is meant in spirit of constructive critique: I realise the people working on this within government, and advising from outside, are working hard to deliver progress often on limited resources and against countervailing pressures, and without their efforts we could be looking at no progress on this issue at all. I remain an idealist, looking to articulate what we should expect from policy, rather than what we can, right now, reasonably expect.)
There are standards, and there are standards
The Algorithmic Transparency Standard is made up of two parts:
- An algorithmic transparency data standard’ – which at present is a CSV file listing 38 field names, brief descriptions, whether or not they are required fields, and ‘validation rules’ (given in all but one case, as ‘UTF-8 string’);
- An algorithmic transparency template and guidance described as helping ‘public sector organisations provide information to the data standard’ and consisting of a Word document of prompts for information that is required by the data standards.
Besides the required/non-required field list from the CSV file, there do not appear to be any descriptions of what adequate or good free text responses to the various prompts, or any stated requirements concerning when algorithmic transparency data should be created or updated (notably, the data standard omits any meta-data about when transparency information was created, or by whom).
The press release describes the ‘formalisation’ route for the standard:
Following the piloting phase, CDDO will review the standard based on feedback gathered and seek formal endorsement from the Data Standards Authority in 2022.
Currently, the Data Standards Authority web pages “recommends a number of standards, guidance and other resources your department can follow when working on data projects”, but appear to stop short of mandating any for use.
The Data Standards Authority is distinct from the Open Standards Board which can mandate data standards for exchanging information across or from government.
So, what kind of standard is the Algorithmic Transparency Standard?
Well, it’s not a quality standard, as it lacks any mechanism to assess the quality of disclosures.
It’s not a policy standard as it’s use is not mandated in any strong form.
And it’s not really a data standard in it’s current form, as it’s development has not followed an open standards process, it doesn’t use a formal data schema language, nor is it on a data standards track.
And it’s certainly not an international standard, as it’s been developed solely through a domestic process.
What’s more, even the template ultimately isn’t all that much of a template, as it really just provides a list of information a document should contain, without clearly showing how that should be laid out or expressed – leading potentially to very differently formatted disclosure documents.
And of course, a standard isn’t really a standard unless it’s adopted.
So, right now, we’ve got the launch of some suggested fields of information that are suggested for disclosure when algorithms are used in certain circumstances in the public sector. At best this offers the early prototype of a paired policy and data standard, and stops far short of CDEI’s recommendation of a “mandatory transparency obligation on public sector organisations using algorithms to support significant decisions affecting individuals”.
Press releases are, of course, prone to some exaggeration, but it certainly raises some red flags for me to see such an under-developed framework being presented as the delivery of a commitment to algorithmic transparency, rather than a very preliminary step on the way.
However, hype aside, let’s look at the two parts of the ‘standard’ that have been presented, and see where they might be heading.
Evaluated as a data specification
The guidance for government or public sector employees using algorithmic tools to support decision-making on use of the standard asks them to fill out a document template, and send this to the Data Ethics team at Cabinet Office. The Data Ethics team will then publish the documents on Gov.uk, and reformat the information into the ‘algorithmic transparency data standard’, presumably to be published in a single CSV or other file collecting together all the disclosures.
Data specifications can be incredibly useful: they can support automatic validation of whether key information required by policy standards has been provided, and can reduce the friction of data being used in different ways, including by third parties. For example, in the case of an effective algorithmic transparency register, standardised structured disclosures could:
- Drive novel interfaces to present algorithmic disclosures to the public, prioritising the information that certain stakeholders are particularly concerned above (see CDEI background research on differing information demands and needs);
- Allow linking of information to show which datasets are in use in which algorithms, and even facilitate early warning of potential issues (e.g. when data errors are discovered);
- Allow stakeholders to track when new algorithms are being introduced that affect a particular kind of group, or that involve a particular kind of risk;
- Support researchers to track evolution of use of algorithms, and to identify particular opportunities and risks;
- Support exchange of disclosures between local, national and international registers, and properly stimulate private sector disclosure in the way the press release suggests could happen;
However, to achieve this, it’s important for standards to be designed with various use-cases in mind, and engagement with potential data re-users. There’s no strong evidence in this case of that happening – suggesting the current proposed data structure is primarily driven by the ‘supply side’ list of information to be disclosed, and not be any detailed consideration of how that information might be re-used as structured data.
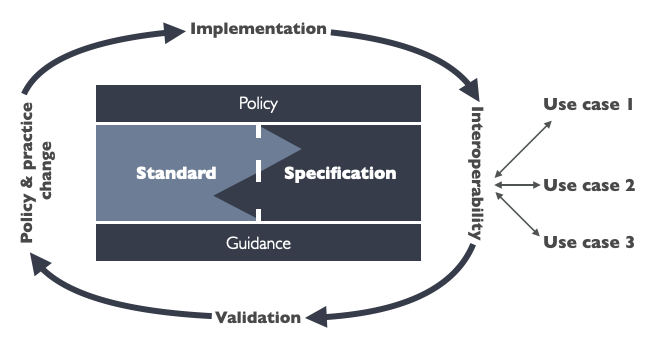
Data specifications are also more effective when they are built with data validation and data use in mind. The current CSV definition of the standard is pretty unclear about how data is actually to be expressed:
- Certain attributes are marked with * which I think means they are supposed to be one-to-many relationships (i.e. any algorithmic system may have multiple external suppliers, and so it would be reasonable for a standard to have a way of clearly modelling each supplier, their identifier, and their role as structured data) – but this is not clearly stated.
- The ‘required’ column contains a mix of TRUE, FALSE and blank values – leaving some ambiguity over what is required (And required by who? With what consequence if not provided?)
- The field types are almost all ‘UTF- string’, with the exception of one labelled ‘URL’. Why other link fields are not validated as URLs does not appear clear.
- The information to be provided in many fields is likely to be fairly long blocks of text, even running to multiple pages. Without guidance on (a) suggested length of text; and (b) how rich text should be formatted; there is a big risk of ending up with blobs of tricky-to-present prose that don’t make for user-friendly interfaces at the far end.
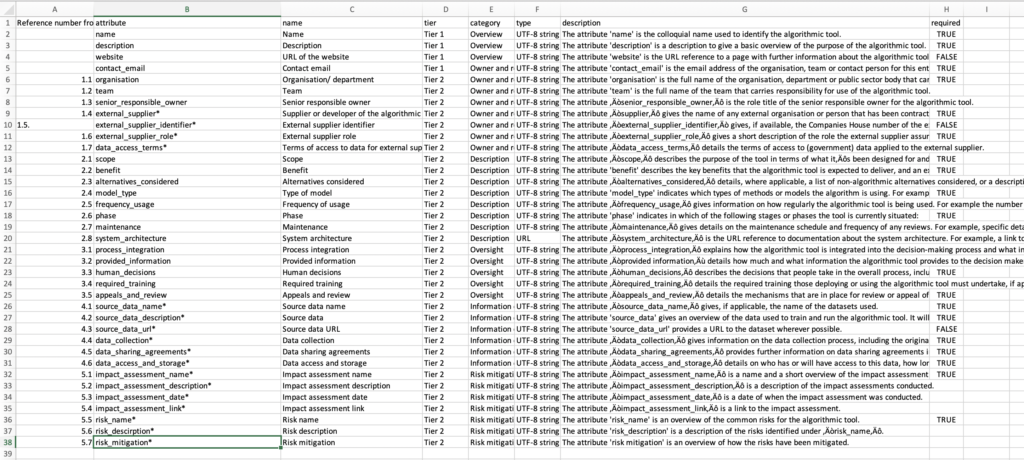
As mentioned above, there is also a lack of meta-data in the specification. Provenance of disclosures is likely to be particularly important, particularly as they might be revised over time. A robust standard for an algorithmic transparency register should properly address this.
Data is more valuable when it is linked, and there are lots of missed opportunities in the data specification to create a better infrastructure for algorithmic transparency. For example, whilst the standard does at least ask for the company registration number of external suppliers (although assuming many will be international suppliers, an internationalised organization identifier approach would be better), it could be also asking for links to the published contracts with suppliers (using Contracts Finder or other platforms). More guidance on the use of source_data_url
to make sure that, wherever a data.gov.uk or other canonical catalogue link for a dataset exists, this is used, would enable more analysis of commonly used datasets. And when it comes to potential taxonomies, like model_type
, rather than only offering free text, is it beyond current knowledge to offer a pair of fields, allowing model_type
to be selected from a controlled list of options, and then more detail to be provided in a free-text model_type_details
field? Similarly, some classification of the kinds of services the algorithm affects using reference lists such as the Local Government Service list could greatly enhance usability of the data.
Lastly, when defined using a common schema language (like JSON Schema, or even a CSV Schema language), standards can benefit from automated validation, and documentation generation – creating a ‘Single Source of Truth’ for field definitions. In the current Algorithmic Transparency Standard there is already some divergence between how fields are described in the CSV file, and the word document template.
There are some simple steps that could be taken to rapidly iterate the current data standard towards a more robust open specification for disclosure and data exchange – but that will rely on at least some resourcing and political will to create a meaningful algorithmic transparency registers – and would benefit from finding a better platform to discuss a standard than a download on gov.uk.
Evaluated as a policy standard
The question “Have we met a good standard of transparency in our use of X algorithm?” is not answered simply by asserting that certain fields of information have been provided. It depends on whether those fields of information are accurate, clearly presented, understood by their intended users, and, in some way actionable (e.g. the information could be drawn upon to raise concerns with government, or to drive robust research).
The current ‘Algorithmic transparency template’ neither states the ultimate goal of providing information, nor give guidance on the processes to go through in order to provide the information requested. Who should fill in the form? Should a ‘description of an impact assessment conducted’ include the Terms of Reference for the assessment, or the outcome of it? Should risk mitigations be tied to individual risks, or presented at a general level? Should a template be signed-off by the ‘senior responsible owner’ of the tool? These questions are all left unanswered.
The list of information to be provided is, however, a solid starting point – and based in relevant consultation (albeit perhaps missing consideration of the role of intermediaries and advocacy groups in protecting citizen interests). What’s needed to make this into a robust policy standard is some sense of the evaluation checklist that needs to be carried out to judge whether a disclosure is a meaningful disclosure or not and some sense of how, beyond pilot, this might become more mandatory and part of the business process of deploying algorithmic systems, rather than simply an optional disclosure (i.e. pilots need to talk about the business process not just the information provision).
Concluding observations
The confusion between different senses of ‘standard’ (gold standard, data standard) can deliver a useful ambiguity for government announcements: but it’s important for us to scrutinise and ask what standards will really deliver. In this case, I’m sceptical that the currently described ‘standard’ can offer the kind of meaningful transparency needed over use of algorithms in government. It needs substantial technical and policy development to become a robust tool of good algorithmic governance – and before we shout about this as an international example, we need to see that the groundwork being laid is both stable, and properly built upon.
On a personal level, I’ve a good degree of confidence in the values and intent of the delivery teams behind this work, but I’m left with lingering concerns that political framing of this is not leading towards a mandatory register that can give citizens greater control over the algorithmic decisions that might affect them.